The Modern Data Problem and Data Mesh
Modern organizations keep data in centralized data platforms. Data get generated by multiple sources and are ingested into this data platform. This centralized data platform serves data for analytics and other data consumption needs for the data consumers in the organization. There are multiple departments in an organization, like sales, accounting, procurement, and many more, that can be visualized as domains. Each of these domains ingests data from this centralized data platform. There are data engineers, data administrators, data analysts, and other such roles that work from the centralized data platform. These data professionals work in silos and have very little knowledge of how these data relate to the domain functionality where they were generated. Managing the huge amount of data is cumbersome, and scaling the data for consumption is a big challenge. Making this data reusable and consumable for analytics and machine learning purposes is a big challenge. Storing, managing, processing, and serving the data centrally is monolithic in nature. Figure 4-1 represents the modern data problem. The data is kept in a monolithic centralized data platform that has limited scaling capability, and data is kept and handled in silos.
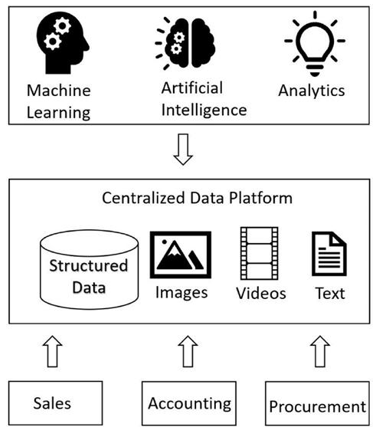
Figure 4-1. Modern data problem
The data mesh solves this modern data problem. It is a well-defined architecture or pattern that gives data ownership to the domains or the departments that are generating the data. The domains will generate, store, and manage the data and expose the data to the consumers as a product. This approach makes the data more scalable and discoverable as the data are managed by the domains, and these domains understand the data very well and know what can be done with them. The domains can enforce strict security and compliance mechanisms to secure the data. By adopting a data mesh, we are moving away from the monolithic centralized data platform to a decentralized and distributed data platform where the data are exposed as products and the ownership lies with the domains. Data are organized and exposed by the specific domain that owns the data generation.